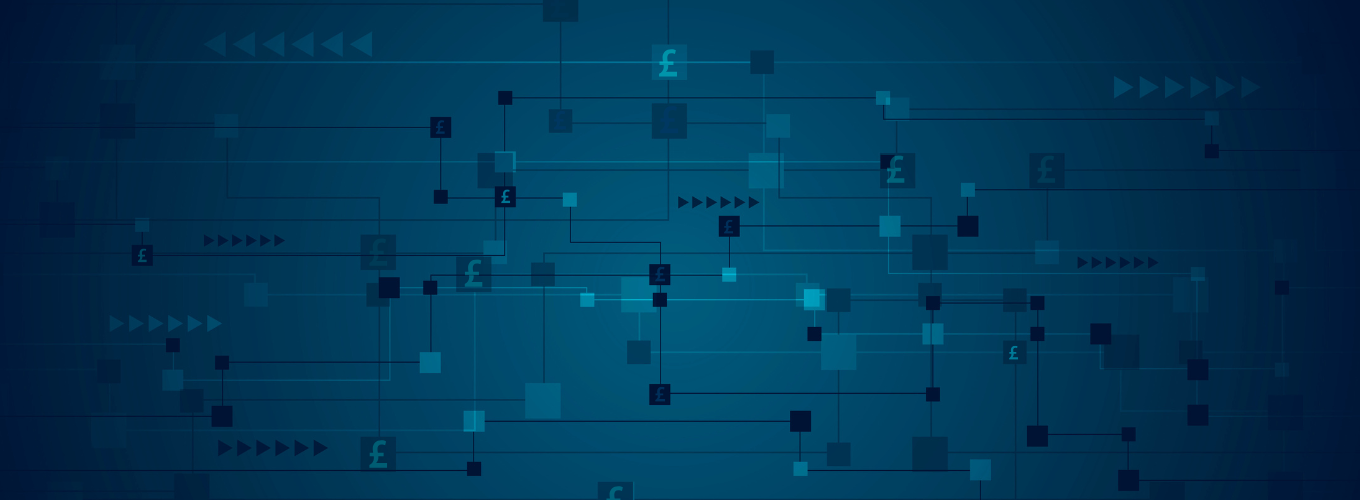
In 2018, the total amount of data created, captured, copied and consumed in the world was 33 zettabytes (around 33 trillion gigabytes), according to a study by IDC and Seagate. This grew to 59 zettabytes in 2020 and is predicted to hit a mind-boggling 175 trillion gigabytes by 2025.
To put these numbers in perspective, one zettabyte is the equivalent of 1,000,000,000,000,000,000,000 bytes. This would hold around 250 billion HD movies or 250 trillion books.
It’s perhaps no surprise then that a recent report from Deloitte has suggested that data collection and analysis will become the basis of all future service offerings and business models by the end of the decade. But as data becomes increasingly complex and abundant, the need for accurate, consolidated and validated data will become more and more essential.
The direct and indirect costs of poor-quality data
A survey of over 500 UK and US business decision makers by Dun & Bradstreet found that nearly two thirds of executives agree that data has helped their businesses mitigate risks, improve the service they provide to their customers and helped identify new opportunities for growth. And yet, in the same survey, well over a third of business leaders admitted that they have struggled with inaccurate data and three fifths worry about the accuracy and completeness of their data going forward.
Whether it’s inaccurate, incomplete, outdated or inconsistent, poor data brings with it significant direct and indirect costs. And with data becoming an increasingly importance aspect of business operations, these challenges are only growing in relevance.
According to research and consulting firm Gartner, poor data quality costs organization an average of $12.9 million (around £9.9 million) every single year. MIT Sloan Management Review, meanwhile, put the price of bad data at around 20% of a company’s revenue.
And that’s just the immediate financial impact.
Poor-quality data wastes time, can result in ineffective decision-making, can damages customer relationships and can put a company’s reputation at risk. This is particularly true in financial services where even the smallest of errors can have huge impacts.
What makes good quality data?
So, what makes good quality data? According to the UK Data Management Association, there are six core pillars of data quality: completeness, uniqueness, consistency, timeliness, validity and accuracy. Let’s take a closer look at what these aspects mean in practice.
Completeness: This describes to whether a dataset contains all the necessary information, without gaps or missing values. The more complete a dataset, the more comprehensive its subsequent analysis is and the better the decisions made from it are.
Uniqueness: This refers to the absence of duplicate records from a dataset, meaning each piece of data is different from the rest. Duplicate data can lead to distorted analysis and inaccurate reporting.
Consistency: Consistency refers to the extent to which datasets are coherent and compatible across different systems or other sets of data. Examples of consistency would be standardised naming conventions, formats or units of measurement across a dataset. Consistency improves the ability to link data from multiple sources.
Timeliness: Straightforwardly, this refers to whether a dataset is up to date and available when needed. This doesn’t necessarily mean the data has to be live to the very moment, but rather that the time lag between collection and availability is appropriate for its intended use. Outdated information can result in obsolete insights.
Validity: This refers to data that conforms to an expected format, type, or range. An obvious example of this would be dates or postcodes. Having validated data helps the smooth running of automated processes and allows data to be used with other sources.
Accuracy: Perhaps the easiest to understand but one of the hardest to get right. Accuracy refers to data which properly represents real-world values or events. High data accuracy allows you to produce analytics than can be trusted and leads to correct reporting and confident decision-making.
Our data quality standards at Raw Knowledge
At Raw Knowledge, we have the highest standards for data quality. We employ a four-eye check every step of the way – from consuming the original data to transforming it into a firm’s required format – and ensure that each piece is stringently validated before we provide it to a client.
Unlike standard data vendors, we specifically work on a given set of financial instruments meaning a firm get can exactly the information they need, in a format they want. This helps ensure the completeness, timeliness and accuracy of our data. We also conduct primary research and compare numerous sources, obtaining original source documentation from issuers and fund managers, to ensure we get things right. All this source material can be provided at request, too, so a firm receives total transparency.